Machine Learning in Finance: Real-World Applications and Challenges
Controlling machine learning in a finance environment requires stakeholders' commitment to creating a strong ethical foundation.
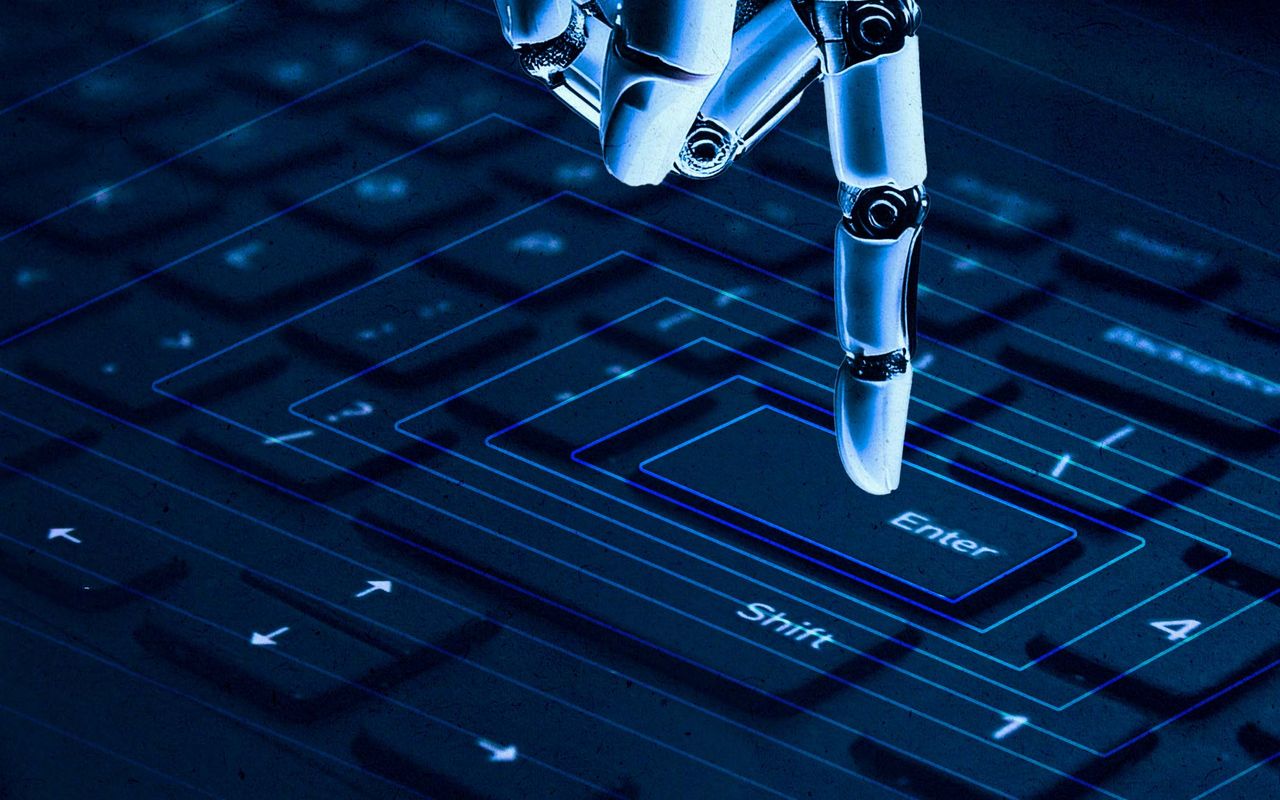
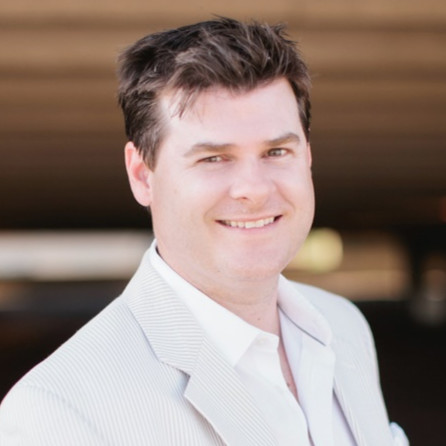
Artificial intelligence (AI) has changed the face of modern finance, bringing the efficiency and security of intuitive decision-making to the business sector.
The use of AI allows various financial institutions to detect real-time fraud, greatly reducing losses and protecting consumers. Through machine learning from AI, massive datasets relating to the stock market are assessed in algorithmic trading to detect real-time trade patterns and execute trades.
Additionally, AI deals with a range of personalized services focusing on financial counseling for consumers and helping them build better money management.
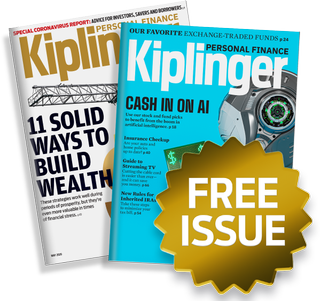
Sign up for Kiplinger’s Free E-Newsletters
Profit and prosper with the best of expert advice on investing, taxes, retirement, personal finance and more - straight to your e-mail.
Profit and prosper with the best of expert advice - straight to your e-mail.
The fundamental transformation, however, comes from the many advances in AI in risk management. There has been an extra push for these institutions to determine borrower creditworthiness, predict cash flow changes and optimize portfolio management; machine learning analyzes vast datasets at a lightning-fast speed, enabling more accurate reasoning and leveraging greater risk management capability with less human error.
These combined tools in finance serve to raise business returns and create a seamless customer-investor experience.
Yet AI finance does present some challenges. The worldwide concern regarding transparency and fairness in AI-based finance institutions springs from bad experiences with issues like data privacy, algorithmic bias and a shifting regulatory ecosystem.
Together, we will identify machine learning applications in finance and discuss both the radical transformation potential and accompanying problems. Controlling machine learning in a finance environment requires stakeholders' commitment to creating a strong ethical foundation.
Key applications of machine learning in finance
Machine learning is changing the face of finance by automating complex processes, increasing security and allowing better decision-making.
Financial institutions can use AI for fraud detection, optimization of trading strategies, personalized customer experience and improved risk management. These technologies enable a much faster and more precise analysis of vast volumes of data, allowing businesses and individuals to make smarter decisions with their funds.
The usefulness of machine learning has mass potential, from real-time fraud detection to AI-powered investment strategies.
Many of these innovations can entirely change how banks, investors and consumers interact with financial services. Some of the highlights in the following sections explain how AI is making an impact and transforming industries through machine learning.
1. Monitoring financial fraud
Financial fraud remains an increasing challenge, but machine learning has broken it down into a much simpler task nowadays. Helping financial institutions detect threats through real-time analysis of transaction patterns allows them in many cases to tap into suspicious activities before they blow up into larger issues.
By recognizing unspecified changes in an individual’s spending behavior, machine learning can help banks and processors reduce risks without inconveniencing the customer. The threat-monitoring process is ongoing, wherein the system continuously learns with each transaction, making it much more efficient over time.
2. Algorithmic trading as an investment hack
Whether dealing with profit or loss, milliseconds can mean the difference between making or breaking a deal in the world of investing. Algorithmic trading primarily relies on AI-driven models to analyze a lump sum of market data, identify trends and execute trades at light speed.
Some new trading platforms help users automate their cryptocurrency trading with customizable, rule-based strategies. This allows for intuitive trading that is less intimidating for the individual newly introduced to trading.
The algorithms work differently than human traders, as they work in harmony to analyze multiple factors at the same time. Thus, emotional or impulsive decision-making is minimized while efficiency and performance are maximized.
Be it high-frequency trading or long-term portfolio optimization, machine learning can allow investors to spot opportunities inside the market and seize them with more precision.
3. Customization in the finance sector
The customer experience has also experienced an AI-driven makeover in the banking sector. Chatbots respond to inquiries and solve problems around the clock, powered by natural language processing.
Personalized financial advice, based on a user’s spending habits and goals, helps individuals make smarter money decisions.
Credit scoring has also become more sophisticated, with AI factoring various financial behaviors beyond traditional credit history and reaching a fairer assessment for borrowers.
Companies no longer have to rely solely on an individual’s credit score, which myriad outside factors can often influence; organizations like mine, whose mission is to bring people’s financial dreams to fruition, can extend their resources to a more diverse set of customers with the help of machine learning.
Challenges for consideration
Applying machine learning in finance in several respects carries quite a few challenges. These include data privacy and security issues, which remain the primary concern requiring financial institutions to comply with guidelines like the General Data Protection Regulation (GDPR) and the California Consumer Privacy Act (CCPA).
AI models are only as good as the data on which they have been trained. If pre-existing biases live in the database, the outcomes will be unfair.
Financial institutions must proactively work on ridding themselves of those biases to provide ethical and equity-based decision-making, especially with regard to lending and credit scoring.
Thus, companies must stay a step ahead in the constantly changing legal milieu. Innovations propelled by AI will dominate the future of the financial sector.
Emerging technologies, including explainable AI and quantum computing, hold the potential to transform and ultimately improve risk fraud detection and trading strategies.
However, a recalibrated sense of responsibility must be undertaken to provide services with transparency, accountability and simplicity. It’s imperative that customers feel as though AI-powered tools are not replacing traditional customer service but rather improving it.
With machine learning on the rise as a competitive tool, organizations must learn to wield this power with discernment.
The financial sector uses machine learning to help detect fraud and for portfolio management, but it still puts money into responsible AI projects to mitigate bias and regulatory concerns.
Many companies have done — and must continue to do — a lot of internal work in transforming their systems to offer finance-oriented efficiency, security and decision-making, working hard to improve their core values and offer their clients the best possible customer service.
Related Content
- Can AI Plan Your Retirement Better Than I Can?
- U.S. Treasury's AI is Catching Tax Cheats and Saving Billions
- What Is AI Investing?
- How Emerging Technologies May Influence the Future of Capital Markets
Disclaimer
The information provided here is not investment, tax or financial advice. You should consult with a licensed professional for advice concerning your specific situation.
Get Kiplinger Today newsletter — free
Profit and prosper with the best of Kiplinger's advice on investing, taxes, retirement, personal finance and much more. Delivered daily. Enter your email in the box and click Sign Me Up.
Clay Bethune is the Founder and CEO at Fintech Finance Group, a firm that specializes in building companies in the fintech sector.
-
How Caregivers for Adults Can Save on Taxes in 2025
Tax Breaks Caring for your parent or spouse can be stressful, but the IRS offers tax breaks for qualifying taxpayers. Here they are.
By Kate Schubel Published
-
The Stock Market Is Selling Off. Here's What Investors Should Do
Investors are fleeing the equities market en masse in response to the Trump administration's "jaw-dropping" tariffs. But the experts say don't panic.
By Karee Venema Last updated
-
How Emerging Technologies May Influence the Future of Capital Markets
Updating legacy systems with next-generation AI can enable fully automated trading, risk assessment and liquidity optimization.
By Jabin Geevarghese George Published
-
Embracing Generative AI for Financial Success
Generative AI has the potential to reshape how we approach learning about and managing our personal finances.
By Rod Griffin Published
-
Why Thoughtful AI Adoption Is the Future of Investment Decision-Making
Taking a proactive approach to AI in investing can lead to more responsible and positive outcomes.
By Dr. Clemen Chiang Published
-
Looking to Adopt AI in Your Finance Org? Consider These Factors First
While AI offers immense potential, its implementation in finance and accounting requires thoughtful planning and precise execution.
By Amrita Choudhary Published
-
Beyond the Hype: A Guide to Investing in AI
As AI technology continues to disrupt markets, its near-limitless utility means it may be a good time to invest in this growing area.
By Clay Bethune Published
-
AI in Accounting: The Future Is Here
With more efficient operations, accountants can now dedicate more energy to strategic planning and providing high-value advisory services to clients.
By Amrita Choudhary Published
-
How Technology and Agile Are Reshaping Customer Experience in Financial Services
The future of financial services will be shaped by the integration of AI and Agile practices.
By Jabin Geevarghese George Published
-
Ethical Implications of AI in Accounting
Even though AI presents multiple benefits in accounting and has been widely adopted, there are various ethical implications attached to its use.
By Amrita Choudhary Published